The risks of AI overuse in today’s localisation landscape

Introduction
It is a story most (if not everyone) of us are familiar with:
You find an interesting company, product or blog article online, go to its website eager to learn more, manually switch the website to your native language and… the magic is gone. The once eye-catching English content suddenly turns bland, boring and bereft of all meaning and emotion.
Sometimes, the content in your native language doesn’t make any sense, is inconsistent in both terminology and style, and some of it even remains in English – be it individual keywords or entire paragraphs.
Back in the day, such low-quality translations used to be a big warning sign for cautious customers. Nowadays, however, this issue is quite prominent all over the internet – especially with young, promising and fast-moving start-ups and scale-ups.
But low translation quality is not limited to small companies with scarce resources: If you look closely enough, you’ll find poorly translated individual pages even on the websites of reputable and well-established global players.
So what happened to the overall translation quality?
In short – over the past 20 years, companies on both client and provider sides became increasingly (over)reliant on Machine Translations.
This development was fuelled by major leaps in translation quality, scalability and cost-efficiency achieved by Neural Machine Translation (NMT) engines such as Google Translate, DeepL or Azure NMT and Large Language Models (LLMs), such as OpenAI’s ChatGPT, Google’s Gemini or Meta’s Llama.
All these systems address many companies’ need for fast and cheap translations at scale, while successfully convincing their customers and, as a matter of fact – the general public, that the output quality is (almost) good enough for publishing.
This, on one hand, led to a downward pressure on Language Service Providers (LSPs) to reduce delivery times and translation rates. On the other hand, however, many LSPs also saw the leaps in Machine Translation technologies as an opportunity to increase their own throughput and grow their margins.
And so, Machine Translations and subsequently Machine Translation Post-Editing (MTPE) kept on growing their market share in the translation industry as a whole. They evolved from a quick win for low-priority content to the go-to solution for pretty much all content types: from low-visibility technical documentation to high-visibility brand campaigns.
Why is overreliance on Machine Translations and MTPE a bad thing?
First things first: Neither Machine Translations, nor the human task of Machine Translation Post-Editing are inherently bad. In fact, they are a great addition in any translator’s toolbox and can be incredibly useful in certain scenarios, which we will discuss later.
And thankfully, most companies understand the risk of using raw and unreviewed Machine Translations output. The negative aspects of MTPE, however, are oftentimes underestimated.
Here’s the clue: Many voices within the translations community argue the decision whether to even use Machine Translation for a specific project should be with the linguists, since they have the expertise and experience needed to determine whether a translatable piece of content is suitable for MTPE or requires a more creative and human approach.
Their argument: “You wouldn’t tell your handyman which tools they’re allowed to use, so why would you tell that to your translator?”
In reality, translations and localisation are still largely viewed as a cost factor and not an investment.
Therefore, the decision to use Machine Translation is usually made by the clients and LSPs and oftentimes is based solely on commercial aspects – budgets and deadlines – irrespective of copy type, priority or visibility. Unsurprisingly, this approach has some serious repercussions on the translation quality further down the line.
The first repercussion is the time pressure usually associated with MTPE tasks. Since MTPE is considered as something that allows the translators to move quicker, the deadlines for MTPE tasks are calculated using much more ambitious metrics than “traditional” human translation.
The expected output rate currently lies between 400-750 words per hour, which is up to three times higher than the output rate for a “traditional” translation, namely 250 words/hour.
This already substantial increase in expected working speed, unfortunately, looks small in comparison to the cost pressure of MTPE. Oftentimes, the MTPE rates offered to freelance linguists can be so low that translators who specialise in MTPE need to reach output speeds of 1.000-1.500 word per hour – or up to 4-6 times the speed of human translations – in order to reach the median net wage of their target language’s country.
As one can imagine, coherent, consistent and compelling translations are anything but a top priority under such conditions.
This creates the downward spiral we’re seeing today: The better Machine Translations become, the more time and cost pressure is put on human translators and the worse the average translation quality becomes, driving even more customers to think Machine Translations are a viable alternative, which, again, leads to more investments into AI and Machine Translations.
Will AI and Machine translations soon become good enough to eliminate human translators and break this spiral? Debatable. What is clear, is that we are not there yet and more and more companies are getting caught in the MTPE trap.
What is also clear is that completely avoiding Machine Translations and MTPE is barely an option anymore, as it leads to slower content creation processes, higher costs and ultimately competitive disadvantages. Luckily, some strategies can help quality-conscious companies to avoid mediocre or outright bad translations where it really matters.
How to avoid the MTPE trap?
To avoid the MTPE trap, it's essential to rethink how and when machine translation is applied. Overreliance on MTPE often results in content that lacks authenticity, consistency, and clarity.
By setting clear content priorities and using smart localisation strategies, companies can harness the advantages of machine translation without sacrificing quality. The following sections outline specific strategies to ensure high-quality localisation tailored to your audience’s needs and expectations.
1. Clear content priorities
Strategy number one is to understand and to categorise your content:
What is your front-window stuff, what can be categorized as “low priority” and, the tricky part – what lies between those extremes. The lines in this categorisation can be very blurry, with lots of different opinions from various stakeholders.
Once you’ve managed to establish clear content prioritisation categories, you can start assigning different translation techniques to them.
A healthy and well thought-through mix of transcreation, localisation, human translation, MTPE and raw Machine Translations will allow you to allocate enough resources to content that has the biggest business impact, while maintaining a satisfactory level of quality for the rest.
How this mix ultimately looks like is as individual as the company defining it. It may not even contain all translation and localisation techniques listed above, as some companies might ditch raw Machine Translations entirely or jump from MTPE straight to fully fletched localisation.
The below image, however, aims to provide you with some inspiration and a rough guideline on what your own mix could look like:

Many of the above content types can also be combined with multiple localisation techniques, depending on a project’s business impact, priority, timeline, target market(s) and many other factors. This is where our second strategy can provide some clarity and flexibility and help to allocate resources more efficiently.
2. Localise smartly
Strategy number two is to utilize such concepts, as “data-driven localisation” and “Minimal Viable Localisation Product” (MVLP).
These two concepts are a product of the emerging notion of Language Operations, or LangOps for short, and utilize similar approaches as DevOps: data-driven decisions and continuous improvements. To demonstrate how data-driven localisation and MVLP can work, let’s take a look at an example – publishing and localising an English-first blog article.
Define localisation thresholds
Before you publish the article, define between 2 and 4 viewer count thresholds and timeframes to reach them. These thresholds will not only help you measure the article’s overall performance, but also serve as triggers for individual localisation steps. For simplicity reasons, we’ll go for 3 thresholds in increments of 1,000 views per step.
Launch the MVLP
Once your English blog article is published, closely monitor its performance in non-English speaking markets. As soon as the combined viewer count from those markets hits 1.000, it’s time to release the MVLP. Here, raw Machine Translations are exceptionally useful, as they allow you to move quickly to address a clear international interest in your content without investing a lot of resources.
At this stage, you can also freely decide whether you want to publish the MVLP for all markets generating the non-English traffic for this blog article or whether you want to focus on just the Top 3, Top 10, Top 25, etc. countries.
MVLP’s first polish
After launching the MVLP, it’s time to take a step away from the original English article and concentrate on its minimally localised versions. The principle remains similar to launching the MVLP: once the viewer count of your minimally localised (or rather translated) article in any of these markets reaches the 1.000 views threshold, it should trigger the next level of localisation.
Depending on your company’s overall localisation policy, market priority and available resources, the MVLP’s first round of polishing can be either post-editing the Machine Translation or substituting it with a fresh human-only translation of the original article.
Final localisation
Before we dive into the last step of this data-driven localisation example, let’s recap first with some imaginary numbers. So far you have:
- Published a blog article in English that generated traffic from 25 non-English speaking countries.
- You have provided a raw Machine translation as Minimal Viable Localisation Product to 10 of those markets.
- Swapped the Machine Translation for a human translation in 5 best-performing markets and the viewer counts just keep on growing.
At this point, you can safely sit back and reap the rewards of your work. Or you can go all in and fully localise the article for the e.g. Top 3 markets. This means engaging with local copywriters, linguists, designers, subject-matter experts, customers and partners to make it truly resonate with the local audience.
This can be achieved by swapping customer quotes to local brands, using country-relevant metrics and statistics, interlinking with local customer success stories, adjusting the formatting style, images and maybe even background colours to local preferences and so on – the possibilities are immense.
What you’ll end up with is a truly localised piece of content that can be actively promoted through Marketing, Sales and Partnerships channels, turned into local marketing campaigns and interlinked in future publications.
The main benefit of this data-driven approach is that you can provide each market with translated or localised content in the most efficient way and per each market’s appetite, without compromising on quality or spreading your resources too thin across too many markets.
Additionally, this approach offers quite a lot of flexibility as you start playing around with thresholds and timeframes. For example, you could lower the localisation threshold for your focus markets while setting the bar higher for countries you are not yet planning to enter actively.
Conclusion
AI- or NMT-powered Machine Translations and Machine Translation Post-Editing have massively changed the translation and localisation industry and will continue to do so for many years to come.
Their ease of use, convenience, low cost, speed and scale make them exceptionally attractive for client companies and Language Services Providers. They can also be a “good enough” (temporary) solution in certain, controlled scenarios, and a great alternative for young companies looking to set foot on the international stage for the first time.
Yet, their benefits quickly start to fade or even turn negative, once you firmly set your sights on long-term, sustainable international growth and start truly prioritising your international customers.
It is at this stage where carefully balanced and highly nuanced localisation, crafted by professional and experienced localisation experts working under fair conditions, can become your key differentiator in a world full of mediocre translations.
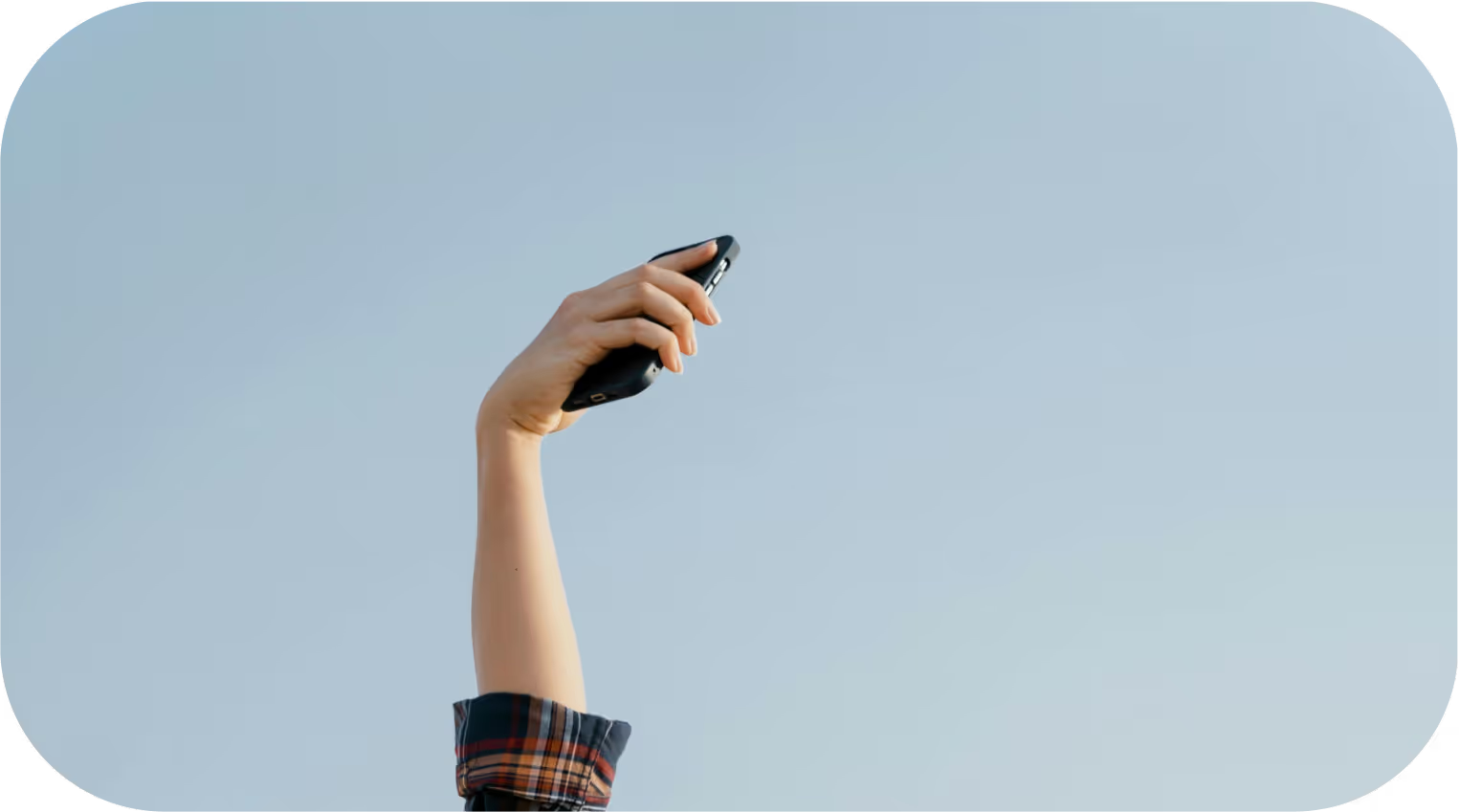
